As we conclude our exploration of AI in networking, it’s time to focus on the practical aspects of implementation. This final blog in our three-part series provides actionable steps and best practices to integrate AI into your networking infrastructure effectively. By following these guidelines, businesses can ensure a smooth transition and maximise the benefits of AI-driven networking.
Assessing your AI readiness
Before diving into AI implementation, it’s crucial to assess your organisation’s AI readiness. This involves evaluating your current infrastructure, identifying gaps, and understanding your specific needs and objectives.
- Infrastructure Audit: Conduct a thorough audit of your existing network assets and infrastructure to determine their suitability for AI integration.
- Skill Assessment: Evaluate the skills and expertise of your IT team. Consider upskilling or hiring talent with AI and machine learning proficiency.
- Data Readiness: Ensure your data is clean, well-organised, and accessible. AI relies on large datasets, so having robust data management practices is essential.
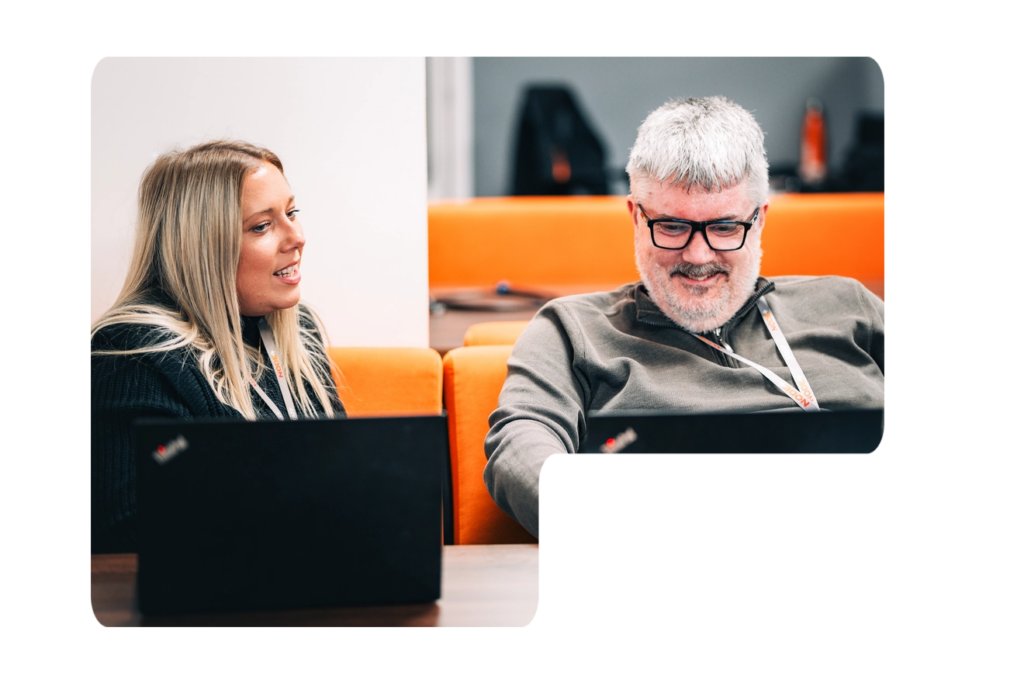
Setting clear objectives
Define clear, measurable objectives for your AI networking initiatives. This will guide your implementation strategy and help track progress.
- Performance Goals: Identify specific performance improvements you aim to achieve, such as reduced downtime, enhanced security, or improved user experience.
- Operational Efficiency: Determine how AI can streamline your operations, automate routine tasks, and reduce manual intervention.
- Customer Experience: Consider how AI can personalise services and enhance customer satisfaction.
Choosing the right AI tools and technologies
Selecting the appropriate AI tools and technologies is critical to the success of your implementation.
- AIOps Platforms: Invest in AIOps platforms that offer comprehensive solutions for network monitoring, predictive analytics, and automated responses.
- HPC and Cloud Solutions: Choose networking systems for high-performance computing (HPC) and cloud solutions that can handle the computational demands of AI applications.
- Edge Computing and IoT: Incorporate edge computing and the Internet of Things (IoT) to collect and process data locally, reducing latency and bandwidth utilisation.
Building a scalable data infrastructure
A scalable data infrastructure is the backbone of AI-driven networking.
- Data Storage: Implement robust and scalable data storage solutions to accommodate growing data volumes.
- Data Integration: Ensure seamless integration of data from various sources, including IoT devices, to provide a comprehensive view for AI analysis.
- Data Security: Maintain stringent data security, encryption, classification, and access practices to protect sensitive information and comply with regulations.
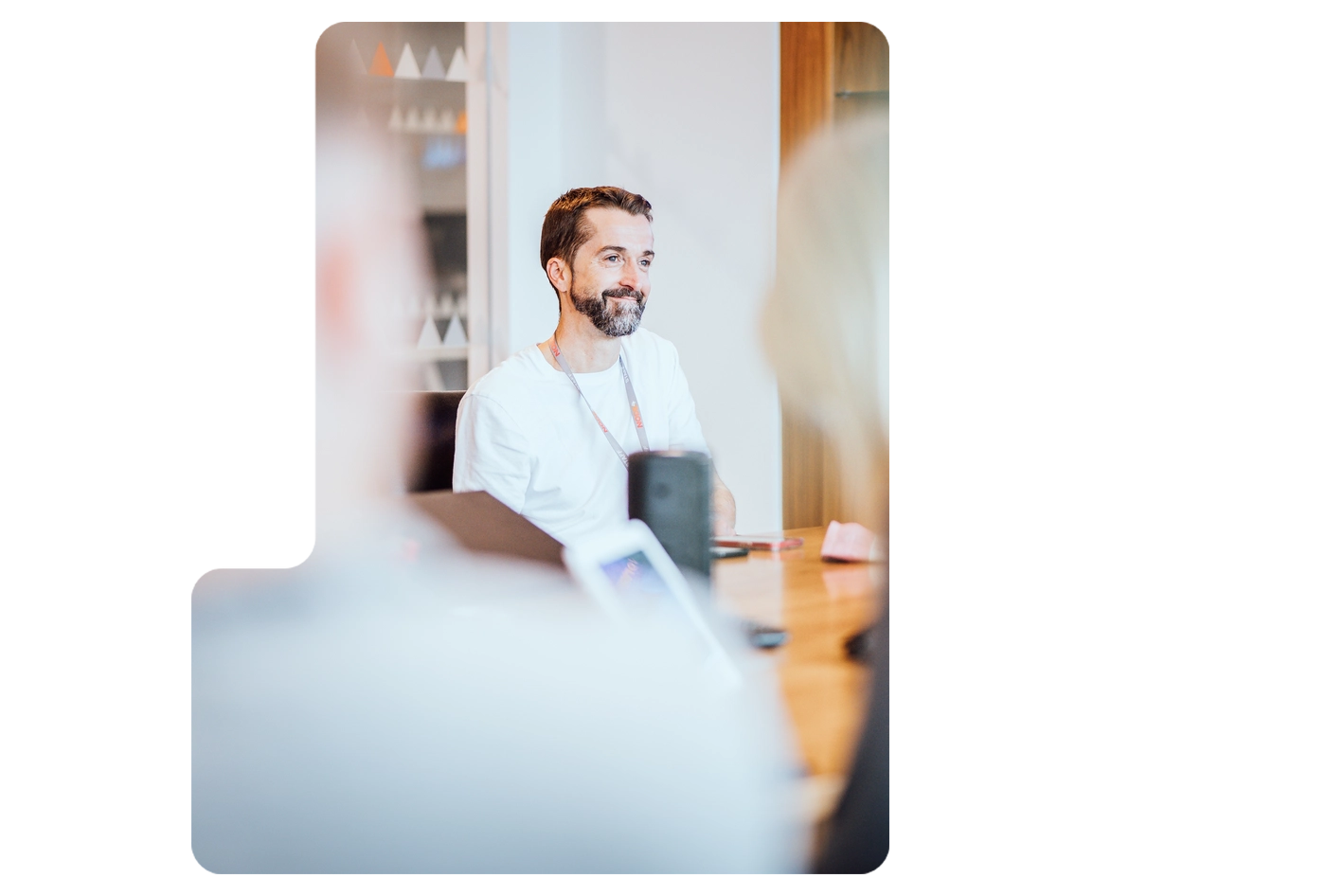
Implementing AI in stages
Implementing AI in networking is a complex process that should be approached in stages.
- Pilot Projects: Start with pilot projects to test AI networking and applications on a smaller scale. This allows you to refine your approach and address any challenges before full-scale deployment.
- Gradual Scaling: Gradually scale up your AI initiatives, expanding to more areas of your network as you gain confidence and experience.
- Continuous Monitoring: Continuously monitor the performance of AI systems, making adjustments as needed to ensure optimal results.
Ensuring robust cybersecurity
AI can significantly enhance cybersecurity, but it also introduces new challenges.
- Threat Detection: Use AI to detect and respond to threats in real-time, protecting your network from cyber-attacks.
- Regular Updates: Keep your AI systems and security protocols up to date to defend against evolving threats, keeping pace with cybercriminals.
- Compliance: Ensure compliance with data protection regulations and industry standards to maintain trust and avoid legal issues.
Fostering a culture of innovation
Successful AI implementation requires a culture of innovation and continuous improvement.
- Encourage Experimentation: Foster an environment where experimentation is encouraged, and failure is viewed as a learning opportunity.
- Collaboration: Promote collaboration between IT, data science/engineering, and business teams to leverage diverse expertise.
- Continuous Learning: Invest in continuous learning and development to keep your team abreast of the latest AI advancements and best practices.
Conclusion: Embrace the future with confidence
As we conclude our three-part series on AI in networking, it’s clear that the integration of AI offers immense potential for transforming network operations and driving business growth. By following the practical steps and best practices outlined in this blog, businesses can navigate the complexities of AI implementation and unlock the full benefits of this transformative technology.
Stay tuned to the Node4 blog and social channels for more insights and guidance on leveraging AI to enhance the productivity of your organisation and achieve your business objectives. Contact us today to learn how we can support you on your AI journey and help you stay ahead in this rapidly evolving landscape.